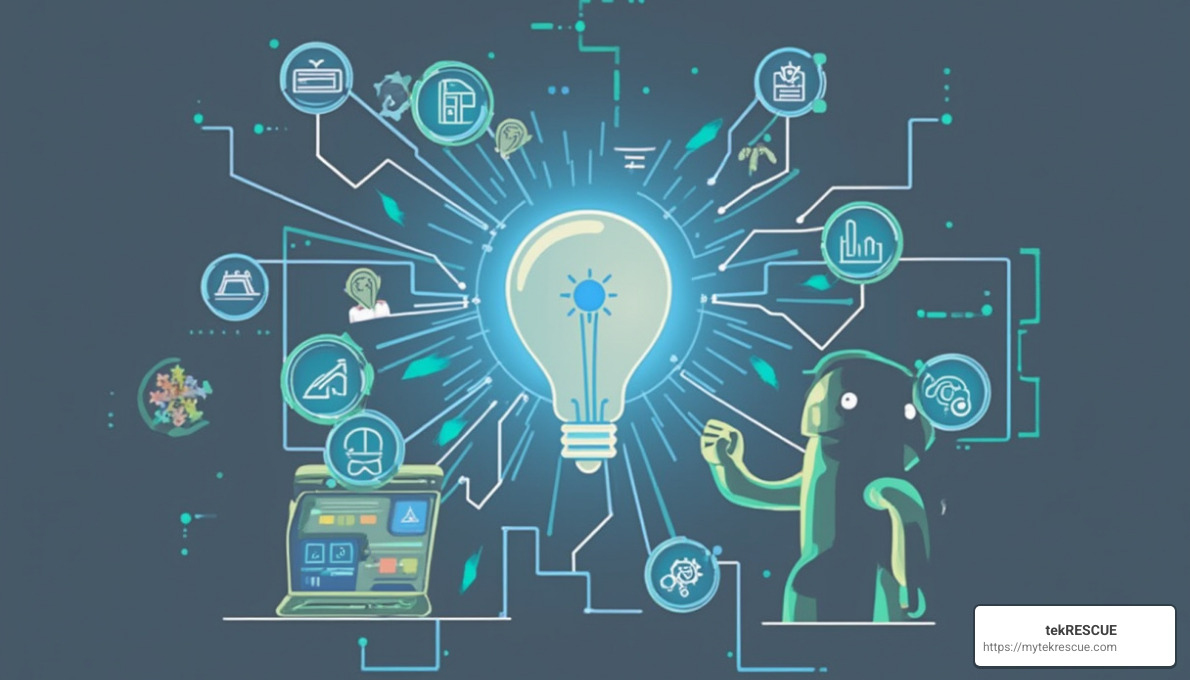
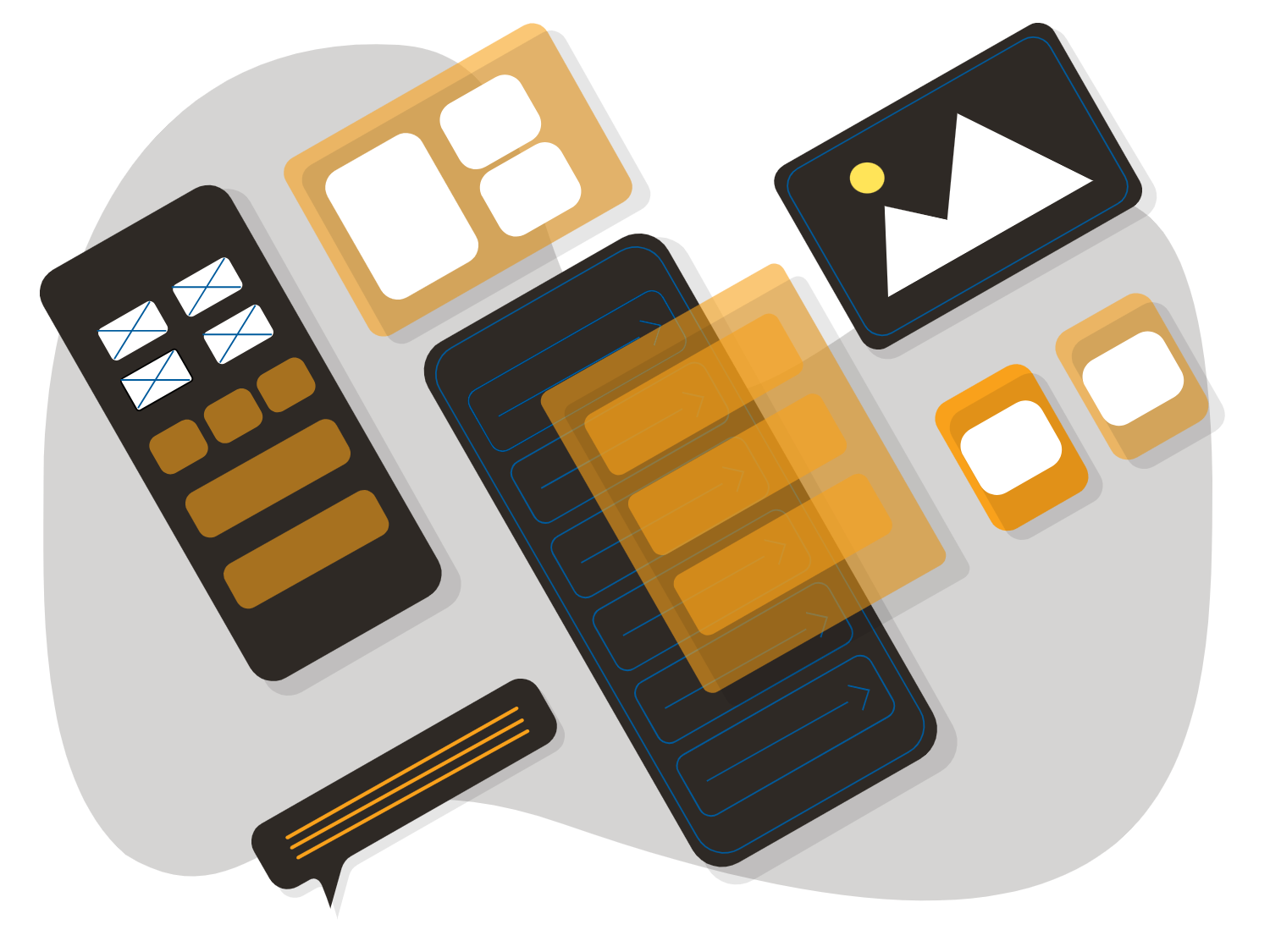
Process Improvement Meets AI – Work Smarter, Not Harder
Revolutionizing Business Efficiency with AI
Have you ever watched your team steer through the same tedious processes day after day and thought, “There must be a better way”? You’re right—there is.
AI for process improvement is changing how businesses operate by intelligently analyzing, optimizing, and automating workflows. It’s like having a tireless efficiency expert who works 24/7, constantly looking for ways to make your operations better.
When we talk about AI for process improvement, we’re talking about moving beyond traditional methods that rely heavily on human analysis. While approaches like Lean and Six Sigma have served businesses well for decades, they often can’t keep pace with today’s data-rich environment. AI changes the game completely.
AI Process Improvement Essentials | Benefits |
---|---|
Automates repetitive tasks | Reduces errors by up to 35% |
Analyzes data patterns to identify bottlenecks | Increases productivity by up to 66% |
Predicts outcomes and suggests optimizations | Cuts process cycle times by 30-50% |
Continuously monitors and adapts workflows | Improves customer satisfaction scores to 9.5/10 |
What makes AI for process improvement truly powerful is its ability to handle complexity that would overwhelm human analysts. While your process improvement team might be able to track a dozen variables, AI can monitor thousands simultaneously, spotting subtle patterns and connections that would otherwise go unnoticed.
As industry expert James McKenna puts it: “Sometimes the best way to improve a complex operation is to make many small adjustments, watch what happens, and repeat.” This iterative approach is where AI truly shines—it can objectively evaluate constraints, test multiple scenarios, and determine optimal solutions much faster than traditional methods.
The magic happens when organizations combine different AI technologies like machine learning, natural language processing, and robotic process automation. These technologies work together to create systems that don’t just follow rules but actually learn from experience and get better over time.
I’ve seen how businesses transform when they implement AI for process improvement. At tekRESCUE, we’ve helped companies move from reactive firefighting to proactive optimization, dramatically cutting costs while boosting both operational efficiency and customer satisfaction.
The best part? You don’t need to overhaul everything at once. Smart implementation starts with identifying high-impact processes where AI can deliver quick wins, building momentum for broader change.
Why Traditional Process Improvement Needs an AI Upgrade
Let’s be honest – methods like Lean, Six Sigma, and Business Process Management (BPM) have been reliable workhorses for decades. They’ve helped countless businesses cut waste, reduce errors, and streamline operations. But in today’s world of overwhelming data and lightning-fast markets, these traditional approaches are starting to show their age – like trying to drink from a fire hose with a coffee cup.
Limits of Lean & Six Sigma in the Data Deluge
Remember when process improvement meant a team with clipboards timing operations and drawing flowcharts? Those days seem quaint now. Traditional methods were created when data was scarce and analysis was done primarily by hand. Today’s reality is dramatically different:
Traditional approaches face serious manual bottlenecks – relying on human observation and interviews that capture only a fraction of what’s really happening in your processes. It’s like trying to understand an entire forest by examining just a few trees.
They also provide limited real-time visibility – typically offering snapshots rather than continuous monitoring. Imagine trying to improve your driving by looking at photos of your car instead of having a dashboard with real-time feedback.
Many organizations struggle with change fatigue as traditional improvement initiatives demand significant human effort to implement and maintain. Your teams might be exhausted from the constant push for incremental improvements that require substantial manual work.
And let’s not forget the headache of legacy system constraints – trying to improve processes that span multiple outdated systems that barely talk to each other.
As Bastian Nominacher wisely noted: “Processes are our greatest lever for value and our fastest lever for change.” Yet traditional methods often fail to fully use this potential in today’s complex business landscape.
The New Wave of Intelligent Process Re-engineering
Business process reengineering is making a comeback – but this time with AI as its superpower. This isn’t your parents’ process improvement; this is change that goes beyond incremental changes:
AI for process improvement enables automation that extends far beyond simple tasks. Today’s AI can handle complex decision-making that previously required human judgment – freeing your team to focus on creative and strategic work.
Modern AI-powered decision intelligence platforms can analyze massive datasets to recommend optimal process adjustments in real-time. Imagine having a brilliant analyst working 24/7, never getting tired, and constantly looking for ways to improve your operations.
Perhaps most exciting is the end-to-end visibility that AI-driven process mining and task mining provide. These tools show you exactly how processes actually flow across systems and departments – revealing bottlenecks and opportunities you never knew existed.
Harvard Business Review captures it perfectly: “AI enables better, faster, and more automated decisions, allowing companies to improve efficiency and produce better outcomes.” This capability is breathing new life into process improvement efforts across industries, making possible changes that once seemed like science fiction.
AI Toolbox for Process Improvement – Core Technologies & How They Work
When it comes to changing your business processes, understanding the right tools is half the battle. AI for process improvement isn’t just a buzzword – it’s a collection of powerful technologies that work together to reinvent how work gets done.
Let’s look at how these technologies compare to traditional approaches:
Technology | Traditional Approach | AI-Improved Approach | Key Benefits |
---|---|---|---|
Process Finding | Manual observation, interviews | Process mining, task mining | Comprehensive, data-driven, real-time |
Analysis | Statistical sampling, manual review | Machine learning pattern recognition | Handles massive datasets, finds hidden patterns |
Decision Making | Rule-based, human judgment | Predictive analytics, recommendation engines | Faster, more consistent, data-informed |
Execution | Manual or basic automation | Intelligent automation, RPA with AI | Handles exceptions, learns and improves |
Monitoring | Periodic audits, reporting | Continuous monitoring, anomaly detection | Real-time insights, proactive alerts |
Machine Learning, NLP & RPA Basics
Think of machine learning as the brain that powers AI for process improvement. Unlike traditional programming that follows fixed rules, ML systems learn from experience and get smarter over time.
When applied to business processes, machine learning excels at prediction – it can forecast when a manufacturing line might fail or when customer demand will spike. It’s also brilliant at classification, automatically sorting documents, requests, or support tickets to the right department without human intervention. And when something unusual happens in your workflow? ML’s anomaly detection capabilities flag it immediately.
Natural Language Processing (NLP) is like giving your AI systems the gift of understanding human communication. This is game-changing for processes that involve documents, emails, or customer interactions. NLP can extract key information from contracts, analyze customer sentiment in support tickets, and even power conversational interfaces that help employees steer complex processes.
Robotic Process Automation (RPA) has evolved from simple screen-scraping to intelligent automation. Modern RPA tools improved with AI can handle variability in processes, adapt to changing environments, and make decisions based on what they’ve learned from previous executions. A major financial institution I worked with cut their loan processing time by 60% by combining RPA with machine learning to handle credit approvals – with fewer errors than their manual process.
Generative & Conversational AI for Knowledge Work
The newest stars in the AI for process improvement universe are generative AI tools that can actually create content and assist knowledge workers in unprecedented ways.
Large Language Models like GPT-4 are changing how we handle information-intensive processes. Rather than starting from scratch, employees can have AI draft communications, summarize lengthy reports, or generate documentation. This isn’t about replacing creative thinking – it’s about eliminating the mundane parts of knowledge work so humans can focus on higher-value activities.
AI-powered chatbots have matured beyond simple FAQ answering. Today’s virtual assistants can guide users through complex workflows, collect information needed for process execution, and provide 24/7 support. One healthcare client implemented a virtual assistant that reduced administrative burden on nurses by 22%, giving them more time with patients.
What’s particularly exciting is how these tools are democratizing process improvement. New AI-based workflow design tools allow non-technical staff to create process flows using natural language, get AI-generated improvement recommendations, and even generate automation code without programming expertise.
The latest PEX Network survey confirms this trend is accelerating – 35% of organizations plan to deploy AI this year, with operations (27%), customer service (24%), and data processing (22%) leading the charge.
Process Mining & Task Mining – Your AI Microscope
You can’t improve what you can’t see, which is why process mining and task mining have become essential tools in the AI for process improvement toolkit.
Process mining acts like an MRI for your business operations. By analyzing event logs from your enterprise systems, it reveals how processes actually flow – not how they’re documented in your procedure manuals. It automatically identifies bottlenecks, compliance issues, and inefficient process paths. One manufacturing client finded that their actual order-to-delivery process had 32 variants, despite having only one “official” process – explaining their inconsistent customer experience.
Task mining complements this view by capturing the desktop-level activities that don’t show up in system logs. It reveals those unofficial workarounds, spreadsheet manipulations, and copy-paste operations that often make up the “hidden factory” in knowledge work. This visibility is invaluable for identifying automation opportunities and training needs.
When used together, these technologies provide a complete picture of how work happens. As McKinsey aptly notes, “Combining process mining and task mining delivers a 360-degree view of operations, overcoming the blind spots of each approach used alone.”
I recently worked with a retail chain that identified potential savings of $200-500 million through process mining. However, they initially captured only about 25% of these savings because they missed all the manual workarounds happening outside their ERP system. By adding task mining, they uncovered these hidden inefficiencies and dramatically increased their improvement results.
For organizations looking to optimize their IT infrastructure, these technologies provide crystal-clear insights into system usage patterns and improvement opportunities. More info about optimizing IT infrastructure
AI for Process Improvement: Benefits & ROI
Let’s face it – implementing new technology is always a question of “what’s in it for me?” When it comes to AI for process improvement, the answer is pretty compelling. The returns aren’t just theoretical – they’re substantial and measurable across your entire business.
Quantifying the Gains of AI for Process Improvement
The numbers tell a powerful story about what happens when AI meets process improvement:
Think about boosting productivity by up to 66% through AI-powered automation. That’s not a typo! Companies implementing these solutions routinely see process cycle times shrink by 30-50%. What could your team accomplish if they suddenly had back half the time they currently spend on routine processes?
One executive at a high-tech manufacturer put it this way: their AI implementation cut their end-to-end activity time by 20-50% while improving efficiency by 10-15%. That’s the difference between struggling to keep up and having breathing room to innovate.
The cost savings are equally impressive. Imagine slashing maintenance costs by 20% through AI-powered predictive maintenance that fixes problems before they become expensive failures. Manufacturing environments typically see up to 50% fewer unplanned outages – which means more production time and happier customers.
Speaking of happy customers – AI for process improvement consistently drives customer satisfaction scores up to 9.5 out of 10. Why? Because processes run faster, more accurately, and deliver more personalized experiences. In today’s world where customer patience is measured in minutes, not days, this advantage is priceless.
Accuracy improvements are another major win. With up to 35% improvement in process accuracy through real-time AI analytics, you’re looking at significantly fewer errors in everything from data entry to document processing. This means less rework, fewer customer complaints, and more consistent compliance with business rules and regulations.
Real-Time Optimization with AI for Process Improvement
The magic of AI for process improvement goes beyond static metrics to enable capabilities that traditional approaches simply can’t match.
With adaptive workflows, your processes become dynamic, automatically adjusting based on current conditions and priorities. One industrial products distributor used this capability to optimize their order-to-delivery process, resulting in 10-15% more on-time, in-full shipments. Imagine what that could mean for your customer retention!
Self-healing processes might sound like science fiction, but they’re very real. AI systems can detect failures or bottlenecks as they happen and automatically implement fixes. No more waiting for someone to notice a problem, escalate it, and scramble for a solution while work piles up.
Anomaly alerts transform how you manage operations. Instead of finding issues during monthly reviews (or worse, when customers complain), AI continuously monitors performance and flags unusual patterns immediately. As one process expert colorfully noted, “AI acts as a ‘tireless analyst’ continuously assessing data to spot inefficiencies.” It’s like having your most detail-oriented employee working 24/7 without ever needing a coffee break.
Perhaps most valuable are the predictive insights that AI provides. Rather than just telling you what went wrong yesterday, AI forecasts what’s likely to happen tomorrow and recommends actions to take today. Transportation companies are already using this capability to predict weather-related disruptions and reroute deliveries before storms hit.
Together, these capabilities create what Accenture aptly calls “intelligent operations” – processes that don’t just execute efficiently but continuously learn and improve themselves. Organizations achieving this state typically realize 40-60% operational efficiency gains through AI-driven scalability.
The bottom line? AI for process improvement delivers ROI that traditional approaches simply can’t match – not just in dollars saved, but in capabilities gained and opportunities created.
AI for Process Improvement in Action: Cross-Industry Use Cases
AI for process improvement isn’t just theoretical – it’s changing real businesses across virtually every industry today. Let’s explore how companies are putting these powerful technologies to work in practical, profit-boosting ways.
Manufacturing – Predictive Maintenance & Quality Control
Manufacturing has acceptd AI with open arms, creating smarter factories and leaner operations:
At Audi, AI-powered visual inspection systems examine welds with superhuman precision. These systems can spot tiny defects a human inspector might miss, all while working 24/7 without fatigue or distraction. The result? Higher quality products and fewer costly recalls.
Predictive maintenance might be the biggest manufacturing win. Rather than waiting for machines to break down or following rigid maintenance schedules, AI analyzes sensor data to predict exactly when equipment needs attention. Toshiba implemented this approach and saw maintenance costs drop by 20% while unplanned downtime plummeted by 50%.
A high-tech manufacturer I worked with implemented AI-driven process optimization throughout their production line. They were amazed when end-to-end activity times shrank by 20-50%. Even better, customer satisfaction jumped by 12-15 percentage points as products arrived faster and with fewer defects.
Healthcare – Patient Flow & Claims Automation
Healthcare organizations are finding AI transforms both clinical and administrative processes:
Triage bots are revolutionizing patient intake. These AI assistants can gather symptoms, check medical history, and direct patients to the right level of care – all before a human gets involved. This ensures the most urgent cases get immediate attention while reducing wait times for everyone.
The paperwork burden in healthcare is enormous, but AI is changing that. Medical coding automation helps ensure accurate billing while freeing up clinical staff to focus on patient care rather than administrative tasks. One hospital network implemented AI for claims processing and cut processing time by 75% while reducing costly errors and denials.
Patient scheduling might seem simple, but it’s incredibly complex when you factor in provider availability, equipment needs, and procedure durations. AI optimization tools are helping healthcare providers see more patients with fewer delays and shorter wait times – a win for both providers and patients.
Finance – Fraud Detection & Credit Decisions
Financial institutions were early AI adopters, and they continue to find innovative applications:
Risk scoring has been transformed by machine learning models that analyze thousands of data points to predict creditworthiness. These models often identify qualified borrowers traditional methods might miss, while also flagging truly high-risk applications. A major bank automated their credit approval workflows with AI and now makes faster decisions with greater accuracy.
Fraud detection systems powered by AI analyze transaction patterns in real-time, identifying suspicious activities that would be impossible for humans to spot across millions of transactions. These systems continuously learn and adapt to new fraud techniques, staying one step ahead of criminals.
The Know Your Customer (KYC) process used to take days or even weeks. Now, AI-powered systems can verify identity documents, check backgrounds, and complete compliance requirements in minutes. This dramatically improves the customer experience while ensuring regulatory requirements are met.
Retail & Supply Chain – Demand Forecasting & Inventory
Retailers and supply chain operators are seeing dramatic improvements with AI:
Zara’s famous fast-fashion model relies heavily on AI-powered demand forecasting. By combining RFID tagging with sophisticated analytics, they can predict what will sell, where, and when with remarkable accuracy. This allows them to minimize inventory while still meeting customer demand.
Last-mile routing optimization uses AI to consider traffic patterns, weather conditions, delivery windows, and vehicle capacity to create the most efficient delivery routes possible. Companies using these tools report fuel savings up to 15% and productivity improvements of 25% – significant numbers in an industry with razor-thin margins.
One retailer I worked with implemented AI to perform a four-way match on contracts, purchase orders, receipts, and invoices. This seemingly simple process improvement uncovered price mismatches that had been draining profits for years. The system paid for itself in less than three months.
AI’s ability to optimize inventory across thousands of products and multiple locations is particularly valuable for businesses with seasonal demand or short product lifecycles. Rather than relying on gut feelings or simple historical averages, these systems consider dozens of factors to place the right products in the right locations at the right time.
For a deeper understanding of how AI is reshaping business processes across industries, the Harvard Business Review analysis provides valuable insights into this change.
Implementing AI-Driven Process Improvement: Step-by-Step Guide
Changing your business processes with AI isn’t as complicated as it might seem. Think of it like renovating a house – you need to understand what you have, create a plan for improvements, and then continually maintain it. Let’s walk through how to make AI for process improvement work for your business.
Step 1 – Find & Map the Current Process
Before jumping into AI solutions, you need a clear picture of how things work today:
Start with some good old detective work. Pull event logs from your business systems like your CRM or ERP software. These digital breadcrumbs reveal how work actually flows through your organization – not just how you think it does.
Process mining tools can analyze these logs to create visual maps of your workflows, showing bottlenecks and variations you might never have noticed. For those desktop activities that don’t show up in system logs, task mining tools can capture what’s happening on employees’ screens.
Talk to the people who use these processes every day. They often have the best insights about pain points and improvement ideas. I’ve found that a casual conversation by the coffee machine sometimes reveals more than formal meetings!
One aerospace company finded through this mapping process that employees were spending over half their time in spreadsheets, with two-thirds of that dedicated to repetitive analyses. That insight immediately highlighted where automation would deliver the biggest bang for their buck.
For those looking to apply these techniques to IT operations specifically, process mapping is essential. More info about AI IT automation
Step 2 – Build the AI Model & Automate
Now that you understand your current process, it’s time to build your AI solution:
First, you’ll need to identify the key data points that will drive your AI model. This is called feature engineering – changing raw data into something your AI can learn from. Think of it as teaching a new employee what information matters most.
Choose the right AI approach for your specific needs. Sometimes a simple rule-based system works best; other times you might need sophisticated machine learning. For many business processes, a combination works wonders.
Training your model is like teaching a new skill – it takes examples and practice. Feed your AI historical process data so it can learn patterns and make predictions. Then test it against data it hasn’t seen before to make sure it’s learned correctly.
Now comes the fun part – putting it all together! You might use RPA bots to handle repetitive tasks, API connections to integrate with your existing systems, and user-friendly interfaces for the parts where humans still need to be involved.
An industrial products distributor combined process and task mining to find that 65% of their orders required manual updates and a third of their invoices needed intervention. By addressing these issues, they open uped $30 million in efficiency gains, $18 million in additional revenue, and $5 million in working capital savings. Not too shabby!
Step 3 – Monitor, Learn & Continuously Improve
Implementing AI for process improvement isn’t a “set it and forget it” affair – it’s more like gardening, requiring ongoing attention to flourish:
Set up dashboards to track your key metrics so you can see if your new AI-powered process is delivering results. Automated alerts can let you know when something’s not working as expected.
Don’t be afraid to experiment! A/B testing different process variations helps you find what works best. Some AI systems can even run these tests automatically, continuously optimizing your processes.
Listen to feedback from everyone involved. Your employees and customers will tell you if things are working better or worse than before. This feedback is gold for refining your approach.
As your AI system gains experience, it gets smarter. Reinforcement learning techniques allow your system to improve based on real-world results, not just historical data.
Once you’ve had success with one process, look for opportunities to apply what you’ve learned elsewhere in your organization. Many businesses find that building a center of excellence helps share best practices across departments.
As Chris Monkman, VP of Product Intelligence at a leading process intelligence company, wisely notes, “Process intelligence makes large language models smarter.” By continuously feeding process data back into your AI models, you create a virtuous cycle of ongoing improvement.
For a deeper dive into how process and task mining work together, check out Better Together research on process mining.
Overcoming Challenges, Governance & Ethics
Implementing AI for process improvement isn’t always smooth sailing. Like any transformative technology, AI brings its own set of problems that need thoughtful navigation. Let’s explore how to address these challenges while keeping your AI initiatives ethical and secure.
Change Management & Skill Building
The human element often determines whether your AI implementation soars or stumbles. Even the most sophisticated AI solution will fail if your team isn’t on board.
Many employees naturally worry that AI will make their roles obsolete. Instead of dismissing these concerns, acknowledge them openly. Show your team how AI for process improvement aims to eliminate the mundane parts of their jobs—not the jobs themselves. Share concrete examples of how AI has freed up employees in similar organizations to do more creative, satisfying work.
Building new skills across your organization is equally important. Consider creating a tiered approach to AI training. Everyone needs basic AI literacy to understand what’s possible (and what’s not). Those directly involved with AI projects need deeper technical skills, while “citizen developers” from business units can become champions who bridge the technical and operational worlds.
Cross-functional collaboration becomes essential when implementing AI for process improvement. The days of IT working in isolation are long gone. Your most successful AI initiatives will bring together process experts who understand the workflows, data scientists who build the models, and IT specialists who integrate the solutions.
One manufacturing company found that their AI implementation faltered until they created mixed teams with representatives from operations, analytics, and IT. Once they established clear roles and shared ownership, adoption accelerated dramatically.
For organizations navigating significant technological change, effective change management makes all the difference. More info about digital change
Ensuring Responsible & Secure AI
As AI for process improvement becomes more central to your operations, responsible governance becomes non-negotiable. This isn’t just about regulatory compliance—it’s about building sustainable, trustworthy systems.
Data privacy and security should be foundational considerations, not afterthoughts. AI systems typically need access to sensitive business and customer data, making robust protection essential. Implement clear governance frameworks that specify who can access what data and for what purpose. Ensure compliance with relevant regulations like GDPR and CCPA, and consider techniques like data anonymization to preserve privacy while enabling AI functionality.
Algorithmic fairness matters too. AI systems can unintentionally perpetuate existing biases or create new ones. Regular auditing of both training data and AI outputs helps identify potential issues before they impact your business. Creating diverse teams to develop and review your AI systems brings varied perspectives that can spot potential problems earlier.
For AI to earn trust, its decisions should be understandable. AI for process improvement works best when people can see why the system made specific recommendations. Choose models that provide explanations for their outputs, and create visualization tools that make AI reasoning transparent. This transparency becomes particularly important when AI is making or influencing decisions that affect customers or employees.
Cybersecurity takes on new dimensions with AI systems. Beyond traditional security concerns, you’ll need to protect your AI models and training data from tampering. Secure the integration points between your AI and other systems, and establish monitoring for adversarial attacks that attempt to manipulate AI outputs.
IBM research highlights that 57% of CEOs worry about data security in AI implementations, while 48% express concern about data accuracy and bias. Addressing these issues proactively builds confidence in your AI for process improvement initiatives.
For deeper insights on managing AI security challenges, explore our guide on Managing AI vulnerabilities.
Frequently Asked Questions about AI for Process Improvement
How much data do we need to start?
When businesses first consider AI for process improvement, one question inevitably comes up: “Do we have enough data?” The good news is you probably have more than you think.
For process mining and findy work, you can typically begin with just 3-6 months of event logs from your key systems. While more data will help capture additional process variations, don’t let perfect be the enemy of good. Even with limited historical information, you can start collecting new data right away to build your foundation.
If you’re looking to implement predictive models, the requirements vary by complexity. Simple models might work reasonably well with just hundreds of examples, while more sophisticated predictions typically need thousands. The more variables you want your AI to consider, the hungrier it gets for data.
I always recommend a practical approach to my clients: start with whatever data you have while simultaneously improving your collection methods. Begin with simpler models that require less training, then evolve as your data resources grow. Many organizations find success with pre-trained models that need less company-specific information to be effective.
As AI expert David Corlette wisely puts it: “AI isn’t useful unless it’s well-designed to incorporate data on the right signals, and unless it’s well-trained.” Focus first on the signals you can reliably measure, then expand your horizons as you grow more sophisticated.
Will AI replace human process experts?
This question keeps many people up at night, and understandably so. However, the real-world experience with AI for process improvement tells a more encouraging story.
Rather than replacement, we consistently see AI complementing human expertise. AI excels at analyzing massive datasets and spotting patterns that would take humans weeks or months to identify. But human experts bring irreplaceable context, judgment, and creative problem-solving abilities to the table. The magic happens when these strengths combine – AI handling the heavy analytical lifting while humans provide the wisdom to apply those insights effectively.
What we’re actually seeing is role evolution, not elimination. Process analysts become AI trainers and supervisors. Technical specialists focus on maintaining and enhancing AI systems. Fascinating cross-functional roles emerge that bridge business operations and AI domains.
Yes, skills do change. Routine analytical tasks increasingly shift to AI systems, freeing human experts to focus on handling exceptions, driving innovation, and making judgment calls that require emotional intelligence. New skills around AI management become increasingly valuable – and often more interesting than the repetitive work being automated.
As research consistently shows, “AI will free human resources to focus on more strategic activities.” In my experience helping businesses implement AI for process improvement, I’ve seen jobs transform into more engaging, higher-value work rather than disappear altogether.
What’s the typical payback period?
When it comes to return on investment, AI for process improvement offers options for both the impatient and the strategic long-term thinker.
For quick wins with payback in just 3-6 months, look to robotic process automation (RPA) for high-volume, rule-based processes. Process mining can rapidly identify immediate improvement opportunities, and simple document processing automation often delivers fast returns.
Medium-term returns (6-12 months) typically come from implementations like predictive maintenance, customer service automation, and supply chain optimization. These require more setup but deliver substantial ongoing value.
Longer-term investments (12+ months) include complex end-to-end process changes, enterprise-wide AI platforms, and advanced cognitive automation. While these take longer to fully realize their value, they often deliver the most transformative results.
Several factors affect how quickly you’ll see returns: process complexity and volume, data quality and availability, integration requirements, and change management needs all play important roles in determining your timeline.
The results can be remarkable. One high-tech manufacturer I worked with reported 20-50% reductions in process time and 10-15% efficiency gains after implementing AI-driven improvements. An industrial products distributor uncovered $30 million in efficiency savings, $18 million in revenue uplift, and $5 million in working capital reduction through their AI initiatives.
The secret to achieving rapid returns? Start with well-defined, high-impact processes where AI can deliver clear value, then expand based on proven success. At tekRESCUE, we help our clients identify these opportunities to ensure they get the fastest possible return on their AI investments.
Conclusion
The marriage of process improvement and artificial intelligence represents one of the most significant opportunities for business change in decades. AI for process improvement goes beyond traditional methodologies by enabling organizations to not just streamline existing processes but reimagine them entirely.
Throughout our exploration, we’ve seen how this powerful combination delivers remarkable results across industries. Companies are achieving productivity gains of up to 66%, error reductions of up to 35%, and process cycle time improvements of 30-50%. Perhaps most telling is the impact on customer experience, with satisfaction scores reaching an impressive 9.5 out of 10 in organizations that have successfully implemented these technologies.
But the true magic of AI for process improvement lies in how it fundamentally changes the nature of organizational evolution. Rather than relying on periodic improvement initiatives that create change fatigue, businesses can now establish continuous, self-optimizing processes that adapt in real-time to changing conditions. Your processes become living systems rather than static workflows.
The journey starts with truly understanding your current processes through advanced techniques like process mining and task mining. These tools reveal what’s actually happening in your organization rather than what you think is happening. From this foundation of truth, you can select the right AI technologies—whether that’s machine learning for prediction, NLP for document processing, RPA for automation, or generative AI for knowledge work—to create meaningful change.
Of course, technology alone isn’t enough. Successful implementation requires thoughtful attention to change management, governance, and ethics. Your people need to understand how AI will improve their work rather than replace them. Your data needs to be handled responsibly. And your AI systems need appropriate oversight to ensure they deliver fair, unbiased results.
At tekRESCUE, we can help businesses across Texas implement AI for process improvement initiatives that deliver measurable results. Our approach combines deep technical expertise with practical business knowledge to ensure that AI implementations drive real value rather than becoming expensive experiments.
Whether you’re just beginning to explore AI or looking to scale existing initiatives, a strategic roadmap is essential. We recommend starting small, focusing on high-impact processes, measuring results rigorously, and expanding based on proven success. This approach minimizes risk while maximizing return on investment.
The future belongs to organizations that can harness the power of AI to work smarter, not harder. By combining the discipline of process improvement with the capabilities of artificial intelligence, you can create operations that are not just efficient but truly intelligent—operations that learn, adapt, and improve continuously.
Ready to explore how AI for process improvement can transform your business? Learn more about our Strategic AI Consulting services and take the first step toward operational excellence. The journey to smarter processes starts with a single conversation.
Table of Contents